We are happy to announce that our ICRG group member, Beste ATAN defended his P.hD. thesis, entitled “New Edge Computıng Offloadıng Methods For Next Generatıon Wıreless Networks” with great success!
The increasing number of mobile applications and massive deployment of connected IoT devices cause computation load due to intensive task requests coming from devices. Computing these tasks by fulfilling the latency requirements of the features or applications are one of the big argument in the next generation of networks. Although mobile or IoT devices provide us with various kinds of functionalities such as entertainment, social networking, or smart home or transportation, but they are not sufficient to process huge data thyself in an effective way due to their storage, computation, and energy limitations. Besides, traditional cloud computing systems that are currently used in the networks are deployed with high distant from the user environments. Therefore, they are not appropriate to provide low-latency required services. One of the promising methods to address the aforementioned problems is offloading the task computing process to edge servers that are in close proximity to the users. This solution is defined by The European Telecommunications Standards Institute (ETSI) and called multi-access edge computing (MEC). According to the task execution requirements, MEC offers more storage and compute power while meeting stringent performance criteria like low latency, low energy consumption, or increased bandwidth. Even if edge computing is a key solution, the task execution decision process between devices and edge servers is a complex decision problem that conventional optimization algorithms struggle to solve to meet the execution requirements. In recent years, the development of learning methods in artificial intelligence (AI) technology helps to create new methods for resolving these kinds of challenging problems. Additionally, both academies and industries have a significant interest to integrate machine learning (ML) and deep learning (DL) methods into next-generation wireless networks. By integrating AI algorithms into edge network environments, it is possible to accelerate the task execution decision-making process and answer the latency-sensitive task execution demands of mobile and IoT devices.
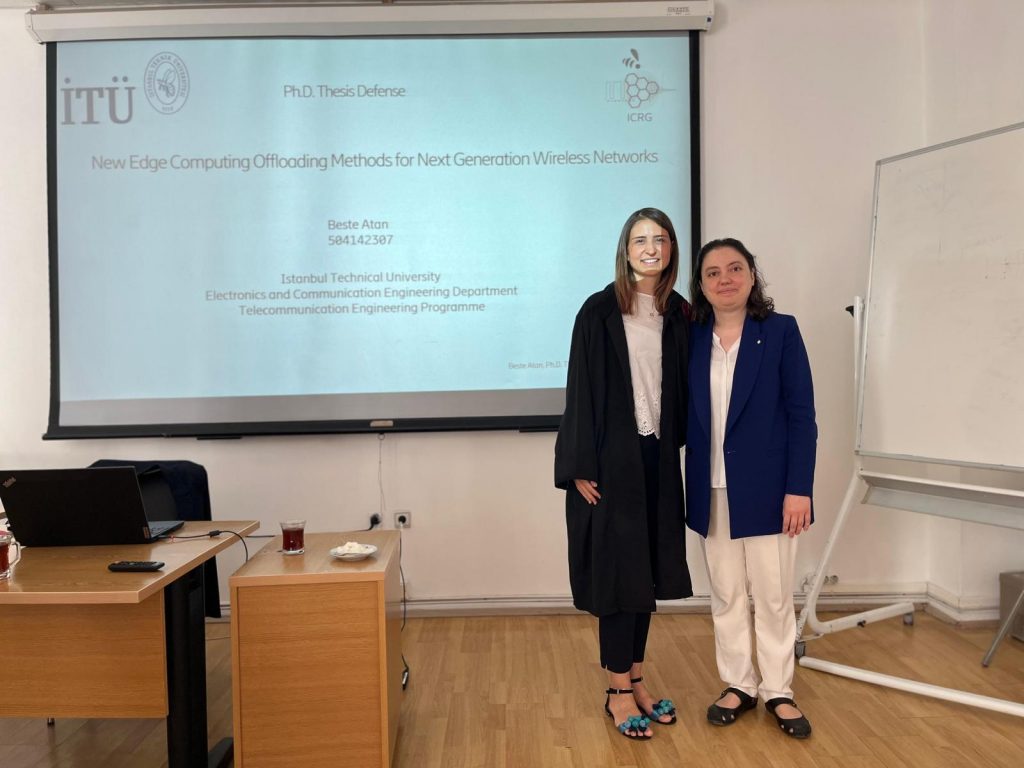
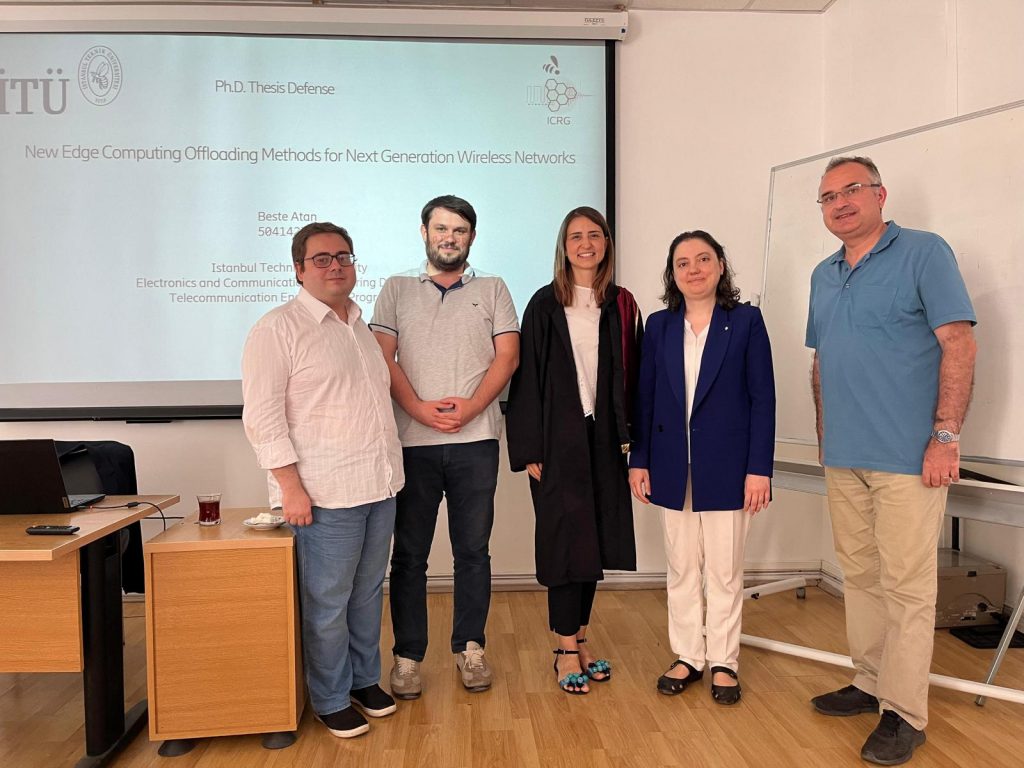
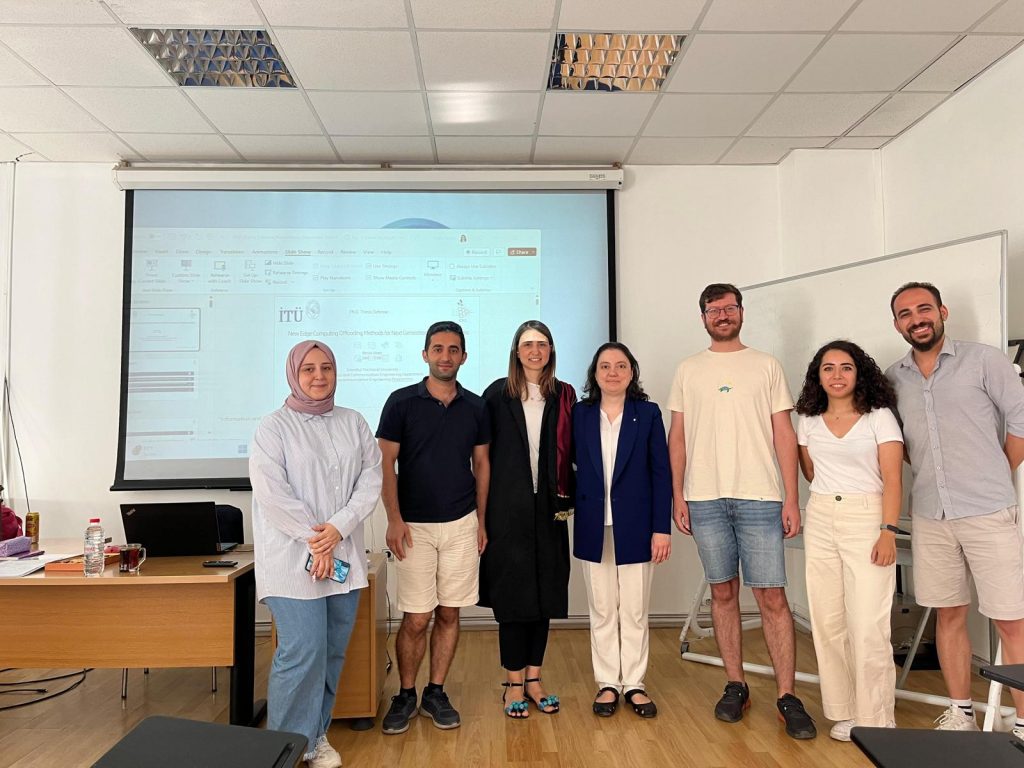
In this thesis, AI-based learning methods are mainly considered for task offloading strategy and we propose an intelligent task execution decision framework to accelerate the edge computation offloading process. In this framework, we take into account the capacity of edge servers, channel conditions, delay and energy consumption of devices, and mobility of users. The system model utilized in this study is built to be feasible for serving a variety of smart connected devices with latency-sensitive applications such as online gaming by consoles, virtual reality (VR) or augmented reality (AR)-based applications, live video streams from unmanned aerial vehicles (UAVs), connected car or smartphone applications. In the first section, task offloading decision methods are investigated for mobile networks by utilizing machine learning (ML) algorithms. In the second section, the study is enhanced to serve multi-device environments for mobile and IoT networks and the AI-empowered task classification framework is designed to respond delay sensitive task execution requests. Comprehensive performance results demonstrate that the proposed AI-empowered framework is substantially fast and precise in the decision-making of the edge computation offloading process while maintaining the quality of user experience compared to conventional optimization methods. We believe that the proposed AI-enabled task classification framework could provide prominent solutions for new applications by running at the network edge.